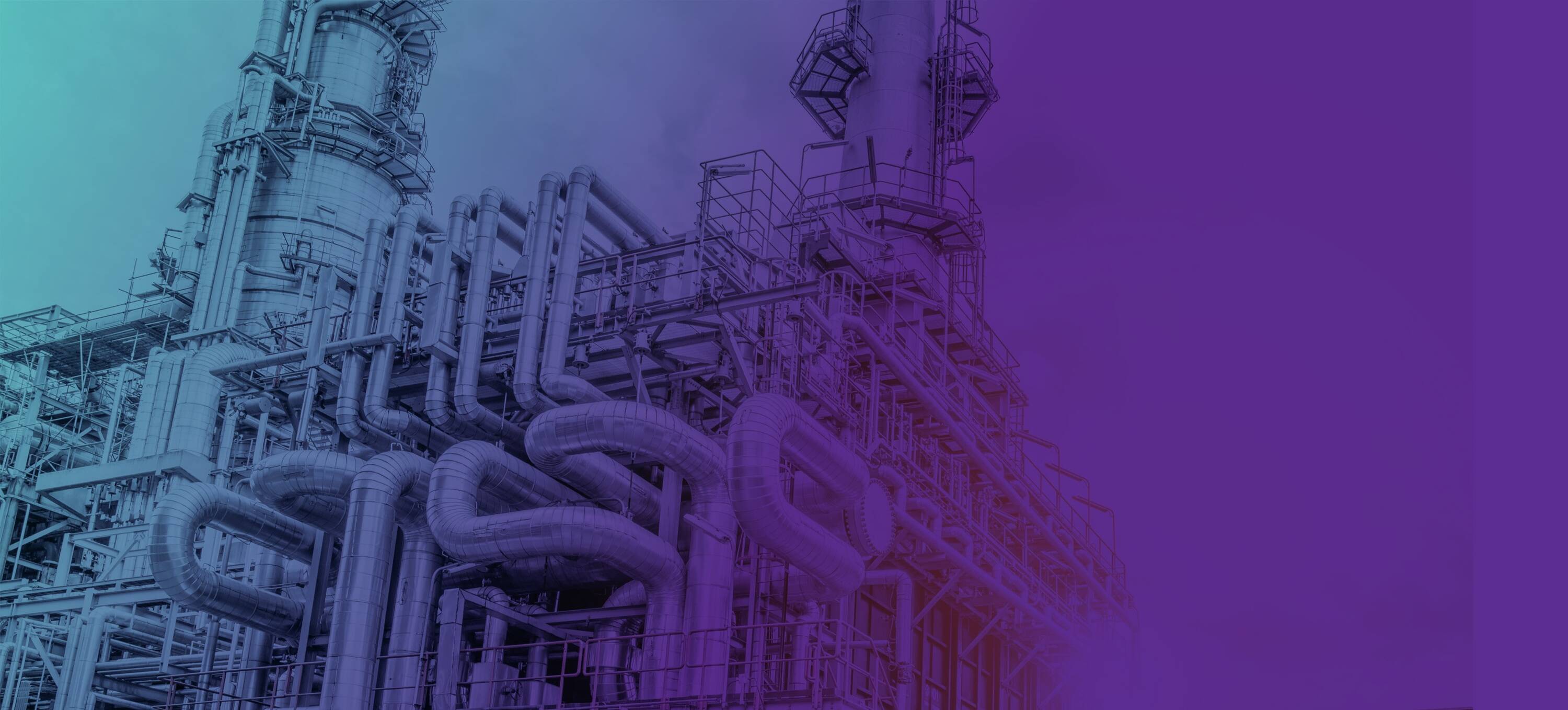
Part 5
What will these factories look like within 10 years? Will robots and artificial intelligence take over, or won’t it work that way? We asked the six participants about their vision for the future.
Maar wat leveren die data nu concreet op? De tweede horde die maakbedrijven nemen, is die data op een overzichtelijke en efficiënte manier aan de medewerkers presenteren.
Guy De Winne (ST Engineering): “De voorbije jaren verzamelden we heel wat data, maar die zat verspreid over verschillende databases, zowel vanuit de productie en machines als uit de ondersteunende diensten. We wilden ervoor zorgen dat alle informatie gecentraliseerd werd in één datacube met verschillende views. Zodat elke medewerker vlot de juiste informatie voor zijn functie uit die centrale database kan halen.”
Voor Lavetan was de verhuis naar een nieuwe, ruimere locatie het startsein voor de digitale transformatie.
Jan Robrechts: “We tekenden één geautomatiseerde waardeketen uit, vanaf het vertrek van het staal bij de klant tot en met de factuur. In onze waardeketen triggert het einde van een procesfase automatisch de daaropvolgende. De intelligentie zit dus versleuteld in het systeem in plaats van in de hoofden van de medewerkers. Die waren immers steeds moeilijker te vinden.
“Onze groei stokte door de schaarste aan labotechnische profielen op de arbeidsmarkt. Maar versleutel je die knowhow zoveel mogelijk in je systemen en processen, dan breek je die talentvijver open. Vandaag heeft ongeveer de helft van onze medewerkers geen labotechnische achtergrond meer nodig.”
Maar het bleef niet bij procesautomatisering alleen. Zo’n vijf jaar geleden startte Lavetan met robotisering, vooral om 24/7 businesscontinuïteit te garanderen. Jan Robrechts: “En sinds een tweetal jaar zitten we in de zogeheten ‘intelligente transformatiefase’. Niet harder, maar vooral nog slimmer werken is nu het motto.”
We tekenden één geautomatiseerde waardeketen uit, vanaf het vertrek van het staal bij de klant tot en met de factuur.”

5. Processen automatiseren
Part 5 – What does the factory of the future look like?

DIGITAL TWIN

Not enough data

The vision of experts
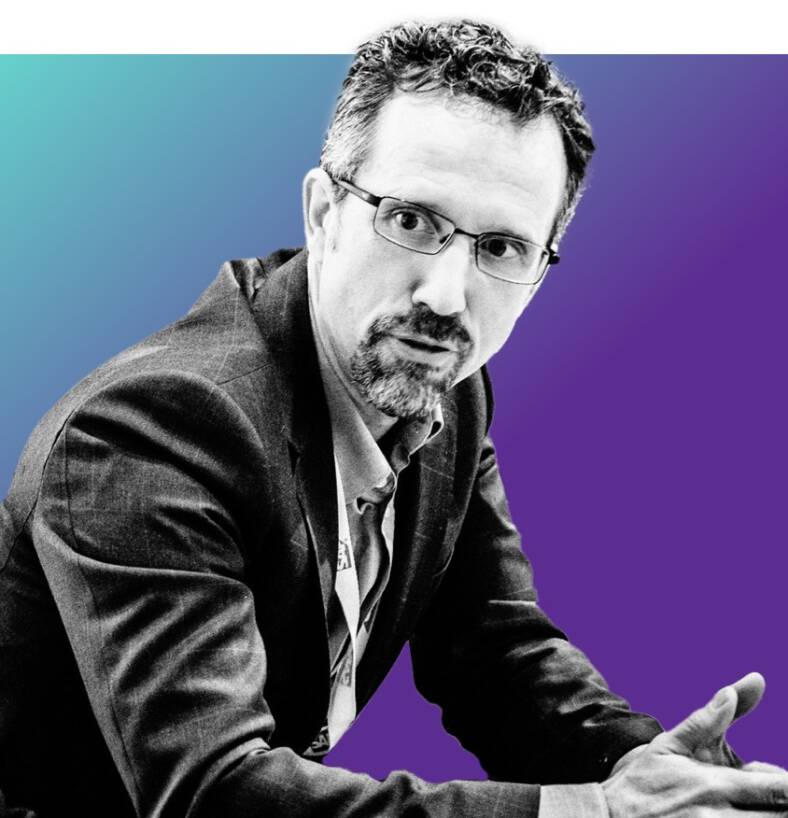
We want to develop our digital twin still further. This involves collecting more data and feeding the machine learning algorithm more and more.”

New technologies do not necessarily apply to every company. For ST Engineering, for example, AI is not (yet) on the agenda.
Guy De Winne: “We don’t have any predictive models yet, because we simply don’t have enough data for this. Our production volumes are too low to really collect a lot of production data. Machine learning and artificial intelligence for data analysis are not feasible for the time being.”
Ontex believes that new technologies are not yet required in order to make a profit.
Jef Monballyu: “We can already become much more efficient using our current technologies alone. We are already learning a lot from our process data these days, but the adjustments still take place manually. Our systems need to become even more self-learning.”
“The availability of more and more digital technologies has accelerated our development, but what will the future hold?”, continues Jef Monballyu. “In any case, we must keep looking out for new things, also beyond the walls of our own business. We've already come this far and the results are excellent, I hear from time to time. But although it’s very tempting sometimes, we should never be satisfied with the status quo.”
Not enough data
Our systems need to become even more self-learning.”

Machine learning, artificial intelligence, big data… The possibilities of new technologies seem endless, but how do the Factories of the Future assess their success in concrete terms? Are these technologies feasible? And are they really as promising as they seem?
What will new technologies bring?
Duracell is already working with a digital twin: a virtual representation of the production process.
Jan Casteels: “By bringing different process parameters together digitally, we can produce a digital battery that is the exact mirror image of the physical battery. We can then experiment with this twin in order to produce the best possible battery. The biggest challenge is finding the right process parameters to feed the digital twin. There are hundreds of parameters, but it isn’t easy to define the impact of each parameter and how they relate to each other. This is a continuous process of improvement that is still ongoing today.”
In the years ahead, Duracell intends to develop this digital twin even further. Jan Casteels: “There is still room for improvement in terms of the quality of the batteries,” says Jan Casteels. “We would also like to fully digitise quality control based on a prediction model. This involves collecting more data and feeding the machine learning algorithm more and more.”
The vision of experts
Geert Jacobs: “The difference in approach between Duracell and ST Engineering lies in the production. Duracell has a huge amount of data points from production, so they have the ability to make a difference with algorithms. ST Engineering makes products with a high degree of complexity, so their challenge is to implement the existing production as efficiently as possible. No two parts are the same, so automating the production makes little sense. In their case, digitisation mainly involves making their production support processes more efficient. Whether a specific kind of new technology is of interest therefore depends to a large extent on the type of production.”
Francis Duvivier believes that small successes can already be achieved with new technologies: “Especially for AI and ML (Machine Learning), some interesting but relatively small-scale case studies exist. Having a strength and a specific focus is the key. In many cases, it is start-ups that specialise in these new technologies. For example, there are players that specialise in particular inefficiencies, detecting anomalies or making predictions. But on a large scale, I’m still not seeing many projects that could be called a true success story.”
Geert Jacobs also confirms this: “Today, I can mainly see potential in AI for imaging. For example, the Kennedy tunnel has smart cameras with AI detection. A good example of how AI can already work in specific applications today.”
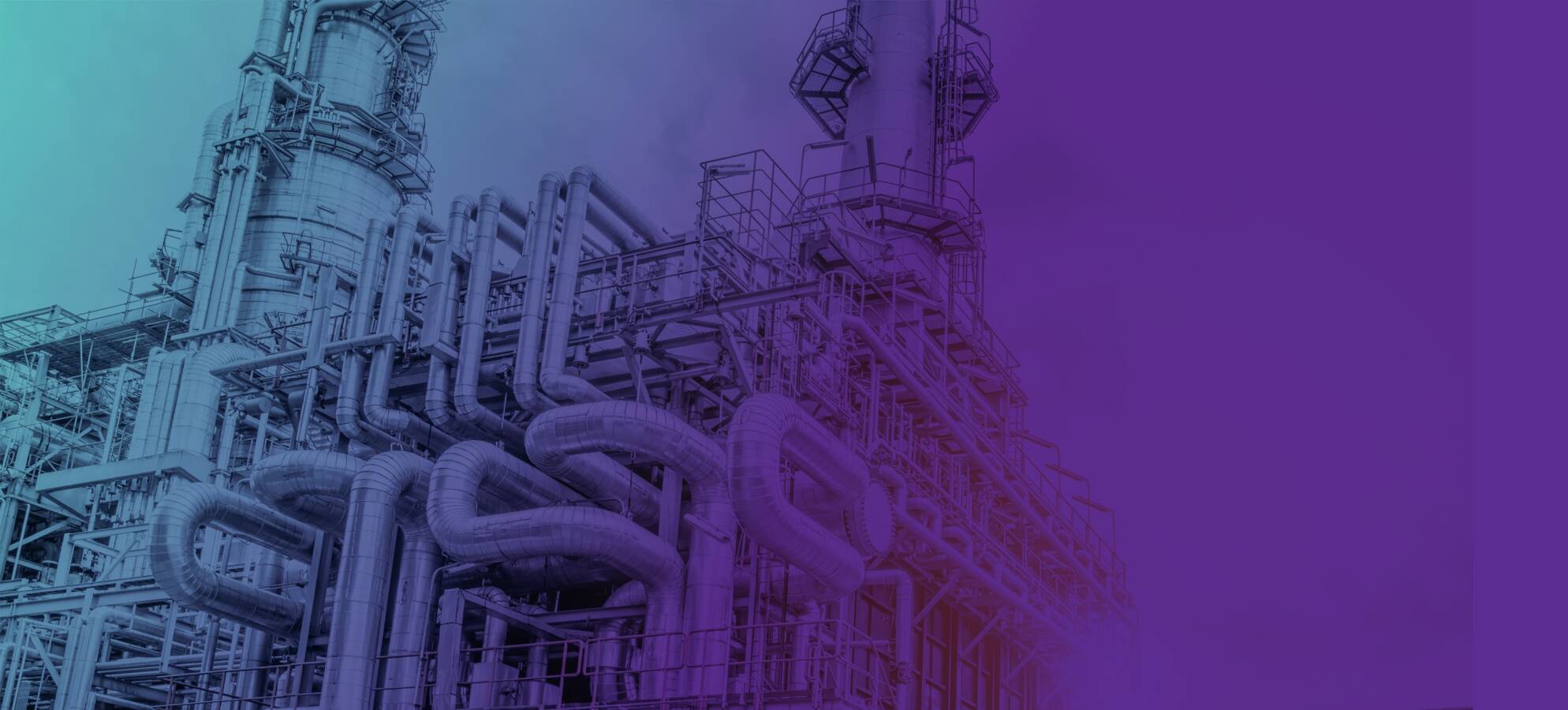
Part 5
What will these factories look like within 10 years? Will robots and artificial intelligence take over, or won’t it work that way? We asked the six participants about their vision for the future.
What will new technologies bring?
Machine learning, artificial intelligence, big data… The possibilities of new technologies seem endless, but how do the Factories of the Future assess their success in concrete terms? Are these technologies feasible? And are they really as promising as they seem?
Duracell is already working with a digital twin: a virtual representation of the production process.
Jan Casteels: “By bringing different process parameters together digitally, we can produce a digital battery that is the exact mirror image of the physical battery. We can then experiment with this twin in order to produce the best possible battery. The biggest challenge is finding the right process parameters to feed the digital twin. There are hundreds of parameters, but it isn’t easy to define the impact of each parameter and how they relate to each other. This is a continuous process of improvement that is still ongoing today.”
In the years ahead, Duracell intends to develop this digital twin even further. Jan Casteels: “There is still room for improvement in terms of the quality of the batteries,” says Jan Casteels. “We would also like to fully digitise quality control based on a prediction model. This involves collecting more data and feeding the machine learning algorithm more and more.”
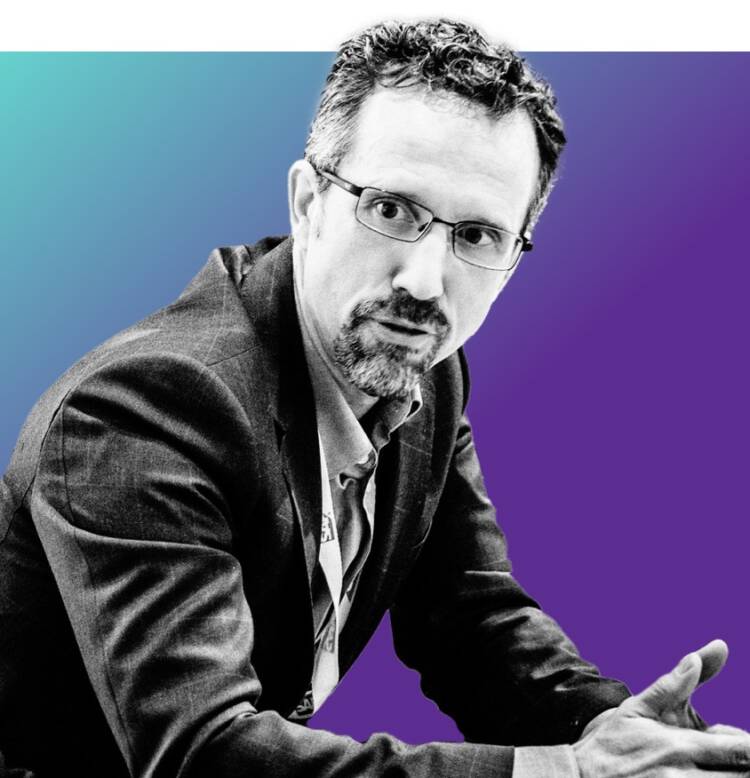
We want to develop our digital twin still further. This involves collecting more data and feeding the machine learning algorithm more and more.”

Not enough data
New technologies do not necessarily apply to every company. For ST Engineering, for example, AI is not (yet) on the agenda.
Guy De Winne: “We don’t have any predictive models yet, because we simply don’t have enough data for this. Our production volumes are too low to really collect a lot of production data. Machine learning and artificial intelligence for data analysis are not feasible for the time being.”
Ontex believes that new technologies are not yet required in order to make a profit.
Jef Monballyu: “We can already become much more efficient using our current technologies alone. We are already learning a lot from our process data these days, but the adjustments still take place manually. Our systems need to become even more self-learning.”
Our systems need to become even more self-learning.”

The vision of experts
“The availability of more and more digital technologies has accelerated our development, but what will the future hold?”, continues Jef Monballyu. “In any case, we must keep looking out for new things, also beyond the walls of our own business. We've already come this far and the results are excellent, I hear from time to time. But although it’s very tempting sometimes, we should never be satisfied with the status quo.”